GeneCentric’s leading-edge rT(I)ME Explorer Platform
The rT(I)ME Explorer Platform is translating tumor biology into robust prognostic and predictive RNA-based gene signatures. Our platform builds upon the pioneering work of co-founders Charles Perou, PhD, and Neil Hayes, MD, MPH, MS, innovators in the molecular characterization and treatment of cancer, and the immunogenomic computational pipelines developed and curated by Benjamin Vincent, MD, and Joel Parker, PhD, founders of Select ImmunoGenomics, which was acquired by GeneCentric in 2019.
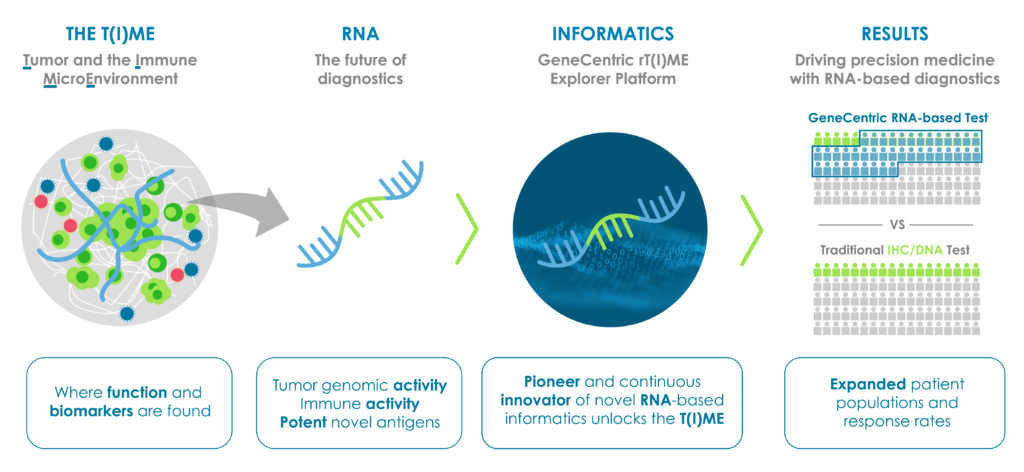
Signature engine & subtypes
A keystone to drug response biomarkers and central to the rT(I)ME Explorer Platform are high-resolution molecular subtypes – inherent genomic signals within a cancer type that are characteristic of its underlying biology. Through advanced computational biology, an expanding portfolio of cancer subtypes and biomarkers accrues within the rT(I)ME Explorer Platform.
Our platform leverages enormous, publicly available gene expression datasets complemented by proprietary data and tools to develop discrete sets of key genes that define cancer subtypes and predict drug response. By reducing the multitude of genes associated with response to discrete key gene set signatures, we enable rapid development of tumor-specific clinical assays. The signatures are platform agnostic, so they are capable of being deployed on established commercial instruments.
Our ability to identify and reduce to practice a select gene set that defines cancer subtypes and predicts drug response underlies our core patents and proprietary position. Our intellectual property includes technology licensed from the University of North Carolina, based on technology developed by Drs. Perou and Hayes, as well as technology developed by GeneCentric.
Immunogenomics
In addition to core tumor biology subtypes, which often include characteristic immune profiles, a deep immunogenomic capability is essential to fully exploring the tumor in the context of its associated microenvironment. The rT(I)ME Explorer Platform is comprised of unparalleled immunogenomic pipelines involving diverse neoantigen prediction, highly curated and proprietary immune gene signatures, and T cell and B cell (TCR/BCR) analysis capabilities. In addition to providing content for deriving predictive biomarkers, the immunogenomic component of the rT(I)ME Explorer Platform is a key target discovery enterprise, particularly with its neoantigen prediction capability.
Our RNA-based Antigen Discovery Immune Oncology (rADIO) informatics platform greatly expands the ability to discover therapeutic tumor antigens, as well as identify biomarkers for predicting immunotherapy response in cancer. rADIO goes beyond the use of gene expression to develop unique response signatures and unlocks underlying RNA sequence information that leads to new drug targets. In addition to discovering new therapeutic antigens, we can apply rADIO to new and existing datasets to identify improved biomarkers of immunotherapy response.
rADIO predicts tumor-specific and tumor-associated antigens from single nucleotide variants (SNVs), insertions and deletions (InDels), fusion events, splice variants, cancer/testis antigens (CTAs), overexpressed self-antigens, viruses, and human endogenous retroviruses (hERVs).
EXpressCT (Expression signatures through circulating tumor signals)
The value of RNA gene expression signatures in tumors is embedded in the fabric of precision medicine but, until recently, the frontier of liquid biopsy has been elusive to these RNA-based signatures. We are deploying a series of approaches based chiefly on fragmentation patterns of cell-free DNA (cfDNA) (fragmentomics) to port diverse gene expression signatures to add to the liquid biopsy armamentarium and improve precision medicine.
TRIUMPH (Transfer Learning RNA Immune Understanding in Microenvironment PHenotype) AI
The tumor microenvironment is a complex set of interactions, and within this intricate matrix lies the potential to unlock new modalities and biomarkers for cancer care. TRIUMPH allows us to capture intricate nuances of this ecosystem.
At the core, TRIUMPH uses transfer learning – a sophisticated AI technique that harnesses pre-existing models and fine-tunes them for specialized tasks. Our focus is on leveraging the wealth of single-cell RNA sequencing (scRNA-seq) data alongside deep learning methods to improve the measurements of specific immune cell types within the tumor microenvironment. These improved measurements can then be used to fuel precision immunotherapy through better predictive algorithms for response to immune therapies.